Matthew Bernacki can predict the future.
It’s a superpower he uses to improve teaching and learning.
Bernacki, Ph.D., the Donald and Justine Tarbet Faculty Scholar at the UNC School of Education, is a pioneer in the development of new ways to observe and analyze how students learn.
Working with a team of researchers, Bernacki uses the methods to uncover new understandings of how students think about and regulate their own learning. Additionally, he and his team use those findings to deliver learning support to those students his models predict will struggle in a class. These supports provide students with additional help and study skill trainings that have been successful in improving their performance.
With funding from the National Science Foundation, Bernacki leads studies that analyze student learning by examining the data generated from college students’ use of online and computer-based instructional tools and materials, including course management systems.
Among the team’s primary objectives: inventing a new method for investigating self-regulated learning.
“The research is clear that students who can self-regulate their learning typically achieve greater academic success,” Bernacki said. “But we need better tools to carry out needed research into which students use self-regulated learning practices, when, and under what conditions. That information can help us understand how their use contributes to greater achievement.
“These tools that we’ve developed — taking advantage of data that students generate when using online learning platforms — hold a great deal of promise in helping us answer important questions that will contribute to gains in teaching and learning,” he said.
The importance of self-regulation
Over two decades, researchers have documented the central role of self-regulation in learning. How a student thinks about their thinking (or, “metacognition”), their own judgments about their ability to learn new material, their planning for how to approach a learning task and the effects of those choices, have been shown to play a large role in determining a student’s success mastering new information.
Theoretical models of self-regulated learning typically describe three phases during learning: a preparatory phase, a performance phase, and an appraisal phase — each of which requires students to monitor their own thinking strategies and make decisions about modifying their strategies based on their own self-evaluation of their success learning new material.
To study self-regulated learning, researchers need to get inside students’ heads. Their methods include asking students to report how they work to learn material, such as “think-aloud protocols” in which students are recorded talking about their experiences and choices as they study. But analyzing the resulting data is labor-intensive, requiring transcription of students’ comments and extensive coding of those comments. As a result, research into self-regulated learning has slowed.
A few learning scientists, including Bernacki and his team, have worked to develop a new method to study self-regulated learning, one that takes advantage of the data generated by students’ use of online resources and course management systems such as Blackboard Learn, Canvas, and Sakai.
By analyzing students’ use of online course materials, Bernacki and his team can track, or “trace,” the behavior of students as they interact with the materials. Such trace data include clicking on and reading teachers’ study guides, use of and performance in practice quizzes, and review of quiz results. Actions such as clicks on buttons, selection of items in dropdown menus, and entry into text fields can trace a student’s work in an online learning environment.
Where Bernacki gets into students’ heads: Inferences from students’ traced behaviors can be matched with processes associated with self-regulated learning. A few examples:
-
- • Accessing syllabi and study guides indicates motivation, forethought, and planning to learn.
-
- • Completing practice quizzes indicates use of active-learning strategies, such as self-testing.
- • Viewing quiz results indicates self-evaluation of performance, effort that contributes to students planning next steps.
Bernacki described the application of trace data in studying self-regulated learning in the book “Handbook of Self-Regulation of Learning and Performance” in a chapter titled “Examining the Cyclical, Loosely Sequenced, and Contingent Features of Self-Regulated Learning: Trace Data and Their Analysis” (Bernacki, 2018).
Using trace data, students can be grouped by their adherence to the phases of self-regulated learning, allowing for examination of students’ varying outcomes and therefore the efficacy of following self-regulated learning practices. For example, some students may engage with study guides and practice quizzes early in a course, while others skip that preparation. Some students work through online practice problems throughout the course, while others don’t. Some go straight to practice quizzes without accessing study guides first. Still others don’t engage much at all with the rich content their instructors provide.
Researchers can use the data to make comparisons between students who follow the phases of self-regulated learning and those who don’t — all to demonstrate whether power rests in the use of self-regulated learning practices.
To help test the validity of their use of trace data to track self-regulated learning, Bernacki and team — as well as other researchers — have conducted studies that compare the use of trace data to track self-regulated learning with previously established methods, such as think-aloud protocols and video capture.
Helping the strugglers
Bernacki began exploring the use of trace data to study self-regulated learning during his dissertation research at Temple University, continuing that work while at the University of Nevada, Las Vegas. He joined the faculty at the UNC School of Education in 2018 to further his research and to teach in the Learning Sciences and Psychological Studies concentration of the Ph.D. program.
The team with which he works includes other learning scientists. At the UNC School of Education that includes Jeffrey A. Greene, the McMichael Family Professor. Team members at UNLV include Jonathan Hilpert, MeganClaire Cogliano, Jennifer Utz, Christy Strong, and Lucie Vosicka. Doctoral students and postdoctoral fellows at the UNC School of Education — including Robert Plumley, Nikki Lobczowski, Mladen Rakovic, Michael Berro, and Shelbi Kuhlmann — also contribute, along with researchers from UNC’s Department of Psychology and Neuroscience.
Bernacki, Greene, and others on the team have collaborated on a number of papers documenting their work using trace data to gauge students’ self-regulated learning.
Among their findings in one study: By tracking the trace data of students in a large introductory-level undergraduate science course, they were able to determine that the most successful students engaged more actively with online resources, activity that predicted subsequent exam performance. The findings regarding timing of students’ use of resources aligned with theory and research on self-regulated learning regarding the importance of early task definition activities as well as the importance of metacognition throughout learning — all of which provides more evidence that students’ use of self-regulated learning practices and higher-order thinking is a powerful tool in online learning environments (Greene, et al., 2021).
They’ve also applied what they’ve learned to create interventions aimed at helping students succeed.
Adding structure to help students
Kelly Hogan had a problem.
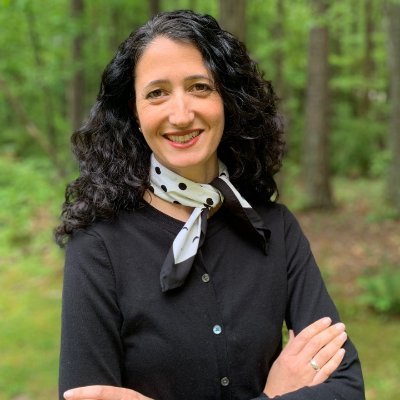
The way she addressed it created opportunities for Bernacki and his team to study students’ learning and to develop an intervention to help students who might struggle in Hogan’s classes.
Hogan, a biology professor and associate dean of instructional innovation at UNC-Chapel Hill, is one of those college professors who teaches large STEM courses, often with more than 400 students. A dozen years ago she was confronted by data that showed a disproportionate number of underrepresented students were failing her Biology 101 course.
Knowing, at that time, nationally only about 40% of students — and only 15% of minoritized students — intending to major in the sciences went on to graduate with a science degree, Hogan sought to transform her teaching so that her courses were not experienced as “weed out” barriers, but as onramps for students seeking to enter STEM fields and careers.
She transformed her teaching from a low-structure format — a traditional lecture-based course that relied on optional readings, lectures, and exams — to a high-structure format that included required online exercises and quizzes throughout the semester, in-class polling and discussion, three exams with a self-reflection after the first exam, a pre-test, and a final exam.
Hogan describes the approach as “inclusive teaching,” as the high-structure format provides more guidance for students who otherwise may not have the background and experience to succeed academically when left largely alone to make their way through a demanding introductory STEM course. The high-structure format worked disproportionately well for Black students — cutting the Black-White performance gap in half — and also for first-generation students (Eddy, et al., 2014).
Now, all professors teaching Biology 101 at UNC use the high-structure format. (Hogan describes this work in her new co-authored book “Inclusive Teaching: Strategies for Promoting Equity in the College Classroom.”)
The use of a high-structure format aligns with calls for reforms from bodies such as the President’s Council of Advisors on Science and Technology that seek wider adoption of active learning practices in early STEM courses as a means to lower barriers to academic success and to reduce disparities within STEM academic programs and fields (Freeman, et al., 2014).
Learning to learn
High-structure, active learning online teaching like that conducted by Hogan and her colleagues requires students to engage strongly in their own learning, using practices described by self-regulated learning models: metacognition, time management, effort regulation, and monitoring and adjusting learning strategies.
Self-regulated learning strategies are especially important in higher education settings as students are expected to learn independently. Research has found that to be especially true in settings where much of a course and its materials are presented in online formats that students are expected to use independently.
High-structure, active learning online teaching also can generate a lot of data — the kind of data that Bernacki and his team can use not only to refine and validate tracking of self-regulated learning, but also to develop interventions to identify and help struggling students.
In a series of published studies, Bernacki and colleagues have demonstrated that trace data can provide early warning signals, identifying students who need help. Students’ patterns of use of a course’s online resources, or lack of use of those resources, provide clues as to who will succeed in the course, and who will not.
“As instructors we didn’t realize just how much data we had for each student and we certainly didn’t know what to do with it,” Hogan said. “Working with Matt and the team, we’re excited to apply ways to use these data to identify students that could benefit from help earlier than we’ve ever identified them.”
In one study, Bernacki and colleagues built a model that could by the end of the third week of a course identify 74% of the students who would end up earning a C or worse on the course’s final exam (Dominguez, et al., 2016). In a more recent study, Bernacki and colleagues were able to cut the warning period to the first two weeks of a course (Cogliano, et al., 2022), the achievement level required for many students to advance in a STEM major or to remain competitive for the careers in health care and research to which they aspire.
Working with data generated by students in the UNC Biology 101 courses, Bernacki, Hogan, and colleagues are studying a new equity-focused layer to their prediction process, creating a set of calculations that seeks to avoid biases common in artificial intelligence and machine learning algorithms. The refined algorithm aims to ensure equitably accurate predictions for those who are under-represented in STEM fields — women, first-generation college students, and Black and Latino students.
Bernacki and colleagues have also built on their findings, developing an intervention that not only provides an early warning to identify students likely to struggle in a course, but also delivers supplemental online resources to help them get on track.
Many universities provide tutoring and other training interventions to assist undergraduate students who lack skills needed to succeed in demanding STEM courses. But these interventions are time-consuming for learners and often rely on face-to-face interaction with facilitators who supply considerable amounts of individualized instruction and feedback. While frequently successful, such personal instruction frequently consumes more time and resources than can be devoted to helping large numbers of students in introductory STEM courses.
To address that problem, Bernacki, among others, has worked to create an online learning skills training program that can be embedded within the context of STEM courses.
An initial version of the program — called “The Science of Learning to Learn” — consists of three modules that introduce students to a set of cognitive strategies that have been proven effective for acquiring knowledge, ensuring its retrieval, and consolidating knowledge into deeper conceptual understandings. The modules can be completed in a few hours, at a time of each student’s choosing, and concurrently with their coursework, thus causing little interruption to students’ work toward primary academic tasks.
The second of the three modules introduces students to the self-regulated learning processes of planning how to approach a learning task, choosing a strategy, and monitoring the effectiveness of their learning strategy.
The learning skills covered in the modules are broadly generalizable toward university coursework, but Bernacki and team designed the modules with relevant content and examples from the STEM courses the students were taking.
Widening pathways into STEM fields
Bernacki’s team conducted randomized control trials to test the effectiveness of “The Science of Learning to Learn” modules. Over the course of three studies, the modules were tested with students taking anatomy and physiology, biology, and algebra. Some students were given the opportunity to use “The Science of Learning to Learn” modules, with others given access to traditional learning supports. (Bernacki, et al., 2020; Bernacki, et al., 2021)
Among the findings:
-
- • First-generation students — who historically perform more poorly in early STEM coursework and exit STEM majors at greater rates — performed better on exams after using the “Science of Learning to Learn” modules than first-generation students in control groups.
-
- • In the algebra course, the “Science of Learning to Learn” students had 8- to 10-point improvement on exams. Because the exam grades were worth 50% of students’ semester grades, the additional points constituted half a letter grade improvement.
- • In the physiology and anatomy course, students using the “The Science of Learning to Learn” modules had a cumulative 10-point gain on exam scores during the semester, equating to one-third of a letter grade, a particularly important improvement for health sciences majors who were required to earn at least a B in the course to continue in the major.
The three studies of the effects of “The Science of Learning to Learn” modules confirm that training a broadly recommended set of cognitive strategies and principles for self-regulated learning provided enduring benefits to hundreds of undergraduate students in courses known to frequently impede students’ progress toward STEM degrees (Bernacki, et al., 2021).
From hours to minutes
Bernacki, working with MeganClaire Cogliano and other colleagues at the University of Nevada, Las Vegas, have demonstrated that a shorter version of the “Science of Learning to Learn” training — one that requires only 15 minutes to complete — is effective in helping students (Cogliano, et al., 2022).
1. Brief explanation of the learning principle + assessment of learning with feedback
2. Description of studies showing practical effect on performance in a college course
3. Worked example illustrating how to use the learning principle in a STEM course
4. Vignette where learning principle is applicable, opportunity to advise a protagonist
5. Prompt to evaluate course resources that afford use of the learning principle
6. Prompt to develop a specific plan how to use the learning principle in the course
That study examined the use of a 15-minute training session covering some basics of self-regulated learning and embedded into the online content of an introductory biology course. Among students who were identified as predicted to fall short of a B in the course, some were given the training session and a control group was not.
The students who received the intervention training had a 12% increase in final exam performance — more than a full letter grade — than the control group. In fact, the performance of the group that had been predicted to fall short of a B but received the “Science of Learning to Learn” intervention matched that of students who had been predicted at the beginning of the course to achieve a B or better.
As the authors state: “A body of work is forming that suggests that brief, digital interventions can remediate learning skills and that the prediction models that classify students as likely to perform poorly can yield insight on the kinds of learning behaviors that might serve as targets for remediation when they align to learning theory.” (Cogliano, et al., 2022).
“More research is needed to explore the use of trace data to inform development of diagnostic prediction models that identify students likely to be in need of learning supports,” Bernacki said.
Additional research is also needed to replicate these findings and to examine how briefer training can influence students’ self-regulated learning processes. Also, fuller randomized control trials with the briefer version of the “Science of Learning to Learn” training are needed to compare its effects with more substantial training methods to examine the effectiveness of differing intensities and targeting of training, Bernacki and colleagues say.
All the while, Bernacki and colleagues continue to develop the “Science of Learning to Learn” program.
Testing of a more engaging, multimedia version is underway in STEM courses. Bernacki also is developing two personalized versions of the “Science of Learning to Learn” training materials that adapt to students’ current ability to skillfully learn and adapt the instruction so students can not only learn skills critical for their STEM courses but can be taught by relatable peers with common backgrounds and identities.
Policymakers and educators are seeking to widen paths of opportunity into STEM fields and careers.
Findings from the work of Bernacki and colleagues demonstrate that while these learning supports provide benefits to all learners, students from populations who typically perform near thresholds that require repeating coursework may be more apt to maintain progress toward STEM degrees when they receive support like that provided by “The Science of Learning to Learn.”
References
Bernacki, M. L. (2018). Examining the cyclical, loosely sequenced, and contingent features of self-regulated learning: Trace data and their analysis. In D. H. Schunk & J. A. Greene (Eds.), Handbook of self-regulation of learning and performance (pp. 370–387). Routledge/Taylor & Francis Group.
Bernacki, M. L., Vosicka, L., & Utz, J. C. (2020). Can a brief, digital skill training intervention help undergraduates “learn to learn” and improve their STEM achievement? Journal of Educational Psychology, 112(4), 765–781. https://doi.org/10.1037/edu0000405
Bernacki, M. L., Vosicka, L., Utz, J. C., & Warren, C. B. (2021). Effects of digital learning skill training on the academic performance of undergraduates in science and mathematics. Journal of Educational Psychology, 113(6), 1107–1125. https://doi.org/10.1037/edu0000485
Bernacki, M. L., Chavez, M. M., & Uesbeck, P. M. (2020). Predicting achievement and providing support before STEM majors begin to fail. Computers & Education, 158. https://doi.org/10.1016/j.compedu.2020.103999
Cogliano, M., Bernacki, M. L., Hilpert, J. C., & Strong, C. L. (2022). A self-regulated learning analytics prediction-and-intervention design: Detecting and supporting struggling biology students. Journal of Educational Psychology. Advance online publication. https://doi.org/10.1037/edu0000745
Dominguez, M., Bernacki, M. L., & Uesbeck, P. M. (2016). Using learning management system data to predict STEM achievement: Implications for early warning systems. Proceedings of the 9th International Conference on Educational Data Mining.
Eddy, S. L., Hogan, K. A. (2014). Getting under the hood: How and for whom does increasing course structure work? Life Sciences Education, Vol. 13, No. 3. https://doi.org/10.1187/cbe.14-03-0050
Freeman, S., Eddy, S. L., McDonough, M., Smith, M. K., Okoroafor, N., Jordt, H., & Wenderoth, M. P. (2014). Active learning increases student performance in science, engineering, and mathematics. Proceedings of the National Academy of Sciences of the United States of America, 111(23), 8410–8415. https://doi.org/10.1073/pnas.1319030111
Greene, J. A. (2018). Self regulation in education. Sage.
Greene, J. A., Urban, C. J., Plumley, R. D., Bernacki, M. L., Gates, K., Hogan, K., Demetriou, C., & Panter, A. (2021). Modeling temporal and contingent self-regulatory processing in a higher education biology course. Learning and Instruction, 72, 101201. https://doi.org/10.1016/j.learninstruc.2019.04.002
Hogan, K. A., and Sathy, V. (2022) Inclusive Teaching: Strategies for Promoting Equity in the College Classroom. West Virginia University Press.
Huang, X., Bernacki, M. L., Ho, D., & Hong. (2022). Examining the role of self-efficacy and online metacognitive monitoring behaviors in undergraduate life science education. Learning and Instruction. https://doi.org/10.1016/j.learninstruc.2021.101577
Lang, C., Siemens, G., Wise, A., & Gasevic, D. (Eds.). (2017). Handbook of learning analytics. SOLAR, Society for Learning Analytics and Research. https://doi.org/10.18608/hla17
Roll, I., & Winne, P. H. (2015). Understanding, evaluating, and supporting self-regulated learning using learning analytics. Journal of Learning Analytics, 21(1), 7-12. https://doi.org/10.18608/jla.2015.21.2
Theobald, E. J., Hill, M. J., Tran, E. Agrawal, S., Arroyo, E. N., Behling, S., & Freeman, S. (2020). Active learning narrows achievement gaps for underrepresented students in undergraduate science, technology, engineering, and math. Proceedings of the National Academy of Sciences of the United States of America, 117(12), 6476-6483. https://doi.org/10.1073/pnas.1916903117
Zimmerman, B. J. (2000). Attaining self-regulation: A social cognitive perspective. In M. Boekaerts, P. R. Pintrich, & M. Zeidner (Eds.), Handbook of self-regulation (pp. 13-39). Academic Press. http://dx.doi.org/10.1016/B978-012109890-2/50031-7
Zimmerman, B. J. (2011). Motivational sources and outcomes of self-regulated learning and performance. In B. J. Zimmerman & D. H. Schunk (Eds.), Handbook of self-regulation of learning and performance (pp. 49–64). Routledge/Taylor & Francis Group.